Forest And Desert
30 January 2025
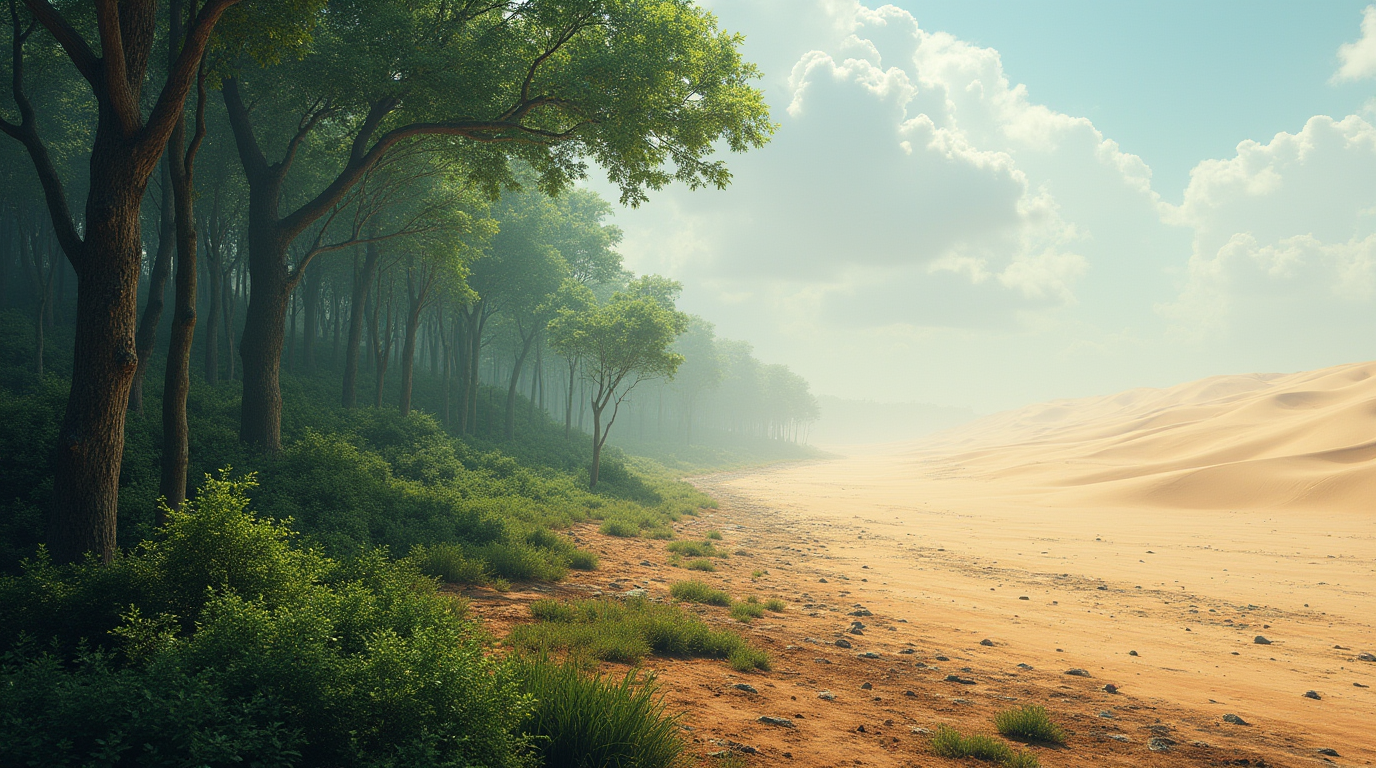
The Forest and the Desert is a metaphor for thinking about software development processes, developed by Beth Andres-Beck and hir father Kent Beck. It posits that two communities of software developers have great difficulty communicating to each other because they live in very different contexts, so advice that applies to one sounds like nonsense to the other.
The desert is the common world of software development, where bugs are plentiful, skill isn't cultivated, and communications with users is difficult. The forest is the world of a well-run team that uses something like Extreme Programming, where developers swiftly put changes into production, protected by their tests, code is invested in to keep it healthy, and there is regular contact with The Customer.
Clearly Beth and Kent prefer The Forest (as do I). But the metaphor is more about how description of The Forest and the advice for how to work there often sounds nonsensical to those whose only experience is The Desert. It reminds us that any lessons we draw about software development practice, or architectural patterns, are governed by the context that we experienced them. It is possible to change Desert into Forest, but it's difficult - often requiring people to do things that are both hard and counter-intuitive. (It seems sadly easier for The Forest to submit to desertification.)
In this framing I'm definitely a Forest Dweller, and seek with Thoughtworks to cultivate a healthy forest for us and our clients. I work to explain The Forest to Desert Dwellers, and help my fellow Forest Dwellers to make their forest even more plentiful.
Further Reading
The best short summary from Beth and Kent is on Kent's Substack. For more depth, take a look at their keynote at Øredev.
Acknowledgements
Kent Beck supplied the image, which he may have painstakingly drew pixel by pixel. Or not.
Cycle Time
4 September 2024
Cycle Time is a measure of how long it takes to get a new feature in a software system from idea to running in production. In Agile circles, we try to minimize cycle time. We do this by defining and implementing very small features and minimizing delays in the development process. Although the rough notion of cycle time, and the importance of reducing it, is common, there is a lot of variations on how cycle time is measured.
A key characteristic of agile software development is a shift from a Waterfall Process, where work is decomposed based on activity (analysis, coding, testing) to an Iterative Process where work is based on a subset of functionality (simple pricing, bulk discount, valued-customer discount). Doing this generates a feedback loop where we can learn from putting small features in front of users. This learning allows us to improve our development process and allows us to better understand where the software product can provide value for our customers. 1
1: It also avoids work being stuck in late activities such as testing and integration, which were notoriously difficult to estimate.
This feedback is a core benefit of an iterative approach, and like most such feedback loops, the quicker I get the feedback, the happier I am. Thus agile folks put a lot of emphasis on how fast we can get a feature through the entire workflow and into production. The phrase cycle time is a measure of that.
But here we run into difficulties. When do we start and stop the clock on cycle time?
The stopping time is the easiest, most glibly it's when the feature is put into production and helping its users. But there are circumstances where this can get muddy. If a team is using a Canary Release, should it be when used by the first cohort, or only when released to the full population? Do we count only when the app store has approved its release, thus adding an unpredictable delay that's mostly outside the control of the development team?.
The start time has even more variations. A common marker is when a developer makes a first commit to that feature, but that ignores any time spent in preparatory analysis. Many people would go further back and say: “when the customer first has the idea for a feature”. This is all very well for a high priority feature, but how about something that isn't that urgent, and thus sits in a triage area for a few weeks before being ready to enter development. Do we start the clock when the team first places the feature on the card wall and we start to seriously work on it?
I also run into the phase lead time, sometimes instead of “cycle time”, but often together - where people make a distinction between the two, often based on a different start time. However there isn't any consistency between how people distinguish between them. So in general, I treat “lead time” as a synonym to “cycle time”, and if someone is using both, I make sure I understand how that individual is making the distinction.
The different bands of cycle time all have their advantages, and it's often handy to use different bands in the same situation, to highlight differences. In that situation, I'd use a distinguishing adjective (e.g. “first-commit cycle time” vs “idea cycle time”) to tell them apart. There's no generally accepted terms for such adjectives, but I think they are better than trying to create a distinction between “cycle time” and “lead time”.
What these questions tell us is that cycle time, while a useful concept, is inherently slippery. We should be wary of comparing cycle times between teams, unless we can be confident we have consistent notions of their stop and start times.
But despite this, thinking in terms of cycle time, and trying to minimize it, is a useful activity. It's usually worthwhile to build a value stream map that shows every step from idea to production, identifying the steps in the work flow, how much time is spent on them, and how much waiting between them. Understanding this flow of work allows us to find ways to reduce the cycle time. Two commonly effective interventions are to reduce the size of features and (counter-intuitively) increase Slack. Doing the work to understand flow to improve it is worthwhile because the faster we get ideas into production, the more rapidly we gain the benefits of the new features, and get the feedback to learn and improve our ways of working.
Further Reading
The best grounding on understanding cycle time and how to reduce it is The Principles of Product Development Flow
Notes
1: It also avoids work being stuck in late activities such as testing and integration, which were notoriously difficult to estimate.
Acknowledgements
Andrew Harmel-Law, Chris Ford, James Lewis, José Pinar, Kief Morris, Manoj Kumar M, Matteo Vaccari, and Rafael Ferreira discussed this post on our internal mailing listPeriodic Face-to-Face
27 February 2024
Improvements in communications technology have led an increasing number of teams that work in a Remote-First style, a trend that was boosted by the forced isolation of Covid-19 pandemic. But a team that operates remotely still benefits from face-to-face gatherings, and should do them every few months.
Remote-first teams have everyone in a separate location, communicating entirely by email, chat, video and other communication tools. It has definite benefits: people can be recruited to the team from all over the world, and we can involve people with care-giving responsibilities. Wasteful hours of frustrating commutes can be turned into productive or recuperative time.
But however capable folks may be at remote working, and however nifty modern collaboration tools become, there is still nothing like being in the same place with the other members of a team. Human interactions are always richer when they are face-to-face. Video calls too easily become transactional, with little time for the chitchat that builds a proper human relationship. Without those deeper bonds, misunderstandings fester into serious relationship difficulties, and teams can get tangled in situations that would be effectively resolved if everyone were able to talk in person.
A regular pattern I see from those who are effective in remote-first work is that they ensure regular face-to-face meetings. During these they schedule those elements of work that are done better together. Remote work is more effective for tasks that require solo concentration, and modern tools can make remote pairing workable. But tasks that require lots of input from many people with rapid feedback are much easier to do when everyone is in the same room. No video-conference system can create the that depth of interaction, staring at a computer screen to see what other people are doing is draining, with no opportunity to pop out for a coffee together to break up the work. Debates about product strategy, explorations of systems architecture, explorations of new ground - these are common tasks for when the team is assembled.
For people to work effectively together they need to trust each other, aware of how much they can rely on each other. Trust is hard to develop online, where there isn't the social cues that can happen when we are in the same room. Thus the most valuable part of a face-to-face gathering isn't the scheduled work, it's chitchat while getting a coffee, and conviviality over lunch. Informal conversations, mostly not about work, forge the human contact that makes the work interactions be more effective.
Those guidelines suggest what the content for a face-to-face should be. Working together is both valuable in its own right, and an important part of team bonding. So we should set a full day of work, focusing on those tasks that benefit from the low-latency communication that comes from being together. We should then include what feels like too much time for breaks, informal chatter, and opportunities to step outside the office. I would avoid any artificial “team building” exercises, if only because of how much I hate them. Those who do gatherings like this stress the value from everyone energized afterwards, and thus able to be more effective in the following weeks.
Remote teams can be formed at large distances, and it's common to see members separated by hours of travel. For such teams, the rule of thumb I would use is to get together for a week every two or three months. After the team has become seasoned they may then decide to reduce the frequency, but I would worry if a team isn't having at least two face-to-face meetings a year. If a team is all in the same city, but using a remote-first style to reduce commuting, then they can organize shorter gatherings, and do them more frequently.
This kind of gathering may lead to rethinking of how to configure office space. Much has been made of how offices are far less used since the pandemic. Offices could well become less of a day-to-day workspace, and more a location for these kinds of irregular team gatherings. This leads to a need for flexible and comfortable team gathering spaces.
Some organizations may balk at the costs of travel and accommodation for a team assembly like this, but they should think of it as an investment in the team's effectiveness. Neglecting these face-to-faces leads to teams getting stuck, heading off in the wrong direction, plagued with conflict, and people losing motivation. Compared to this, saving on airplanes and hotels is a false economy.
Further Reading
Remote-first is one form of remote work, I explore the different styles of remote working and their trade-offs in Remote versus Co-located Work.
At Thoughtworks, we learned the importance of regular face-to-face gatherings for remote teams when we first started our offshore development centers nearly two decades ago. These generated the practices I describe in Using an Agile Software Process with Offshore Development.
Remote work, particularly when crossing time zones, puts a greater premium on asynchronous patterns of collaboration. My colleague Sumeet Moghe, a product manager, goes into depth on how to do this in his book The Async-First Playbook
Atlassian, a software product company, has recently entirely shifted to remote working, and published a report on its experiences. They have learned that it's wise for teams to have a face-to-face gathering roughly three times per year. Claire Lew surveyed remote-first teams in 2018, noting that a quarter of their respondents did retreats “several times a year”. 37Signals has operated as a remote-first company for nearly two decades and schedules meetups twice a year.
Acknowledgements
Alejandro Batanero, Andrew Thal, Chris Ford, Heiko Gerin, Kief Morris, Kuldeep Singh, Matt Newman, Michael Chaffee, Naval Prabhakar, Rafael Detoni, and Ramki Sitaraman discussed drafts of this post on our internal mailing list.
Legacy Seam
4 January 2024
When working with a legacy system it is valuable to identify and create seams: places where we can alter the behavior of the system without editing source code. Once we've found a seam, we can use it to break dependencies to simplify testing, insert probes to gain observability, and redirect program flow to new modules as part of legacy displacement.
Michael Feathers coined the term “seam” in the context of legacy systems in his book Working Effectively with Legacy Code. His definition: “a seam is a place where you can alter behavior in your program without editing in that place”.
Here's an example of where a seam would be handy. Imagine some code to calculate the price of an order.
// TypeScript
export async function calculatePrice(order:Order) {
const itemPrices = order.items.map(i => calculateItemPrice(i))
const basePrice = itemPrices.reduce((acc, i) => acc + i.price, 0)
const discount = calculateDiscount(order)
const shipping = await calculateShipping(order)
const adjustedShipping = applyShippingDiscounts(order, shipping)
return basePrice + discount + adjustedShipping
}
The function calculateShipping
hits an external service, which is slow (and
expensive), so we don't want to hit it when testing. Instead we want to
introduce a stub, so we can provide a canned and deterministic response for
each of the testing scenarios. Different tests may need different responses
from the function, but we can't edit the code of
calculatePrice
inside the test. Thus we need to introduce a seam around the call
to calculateShipping
, something that will allow our test to
redirect the call to the stub.
One way to do this is to pass the function for
calculateShipping
as a parameter
export async function calculatePrice(order:Order, shippingFn: (o:Order) => Promise<number>) { const itemPrices = order.items.map(i => calculateItemPrice(i)) const basePrice = itemPrices.reduce((acc, i) => acc + i.price, 0) const discount = calculateDiscount(order) const shipping = await shippingFn(order) const adjustedShipping = applyShippingDiscounts(order, shipping) return basePrice + discount + adjustedShipping }
A unit test for this function can then substitute a simple stub.
const shippingFn = async (o:Order) => 113 expect(await calculatePrice(sampleOrder, shippingFn)).toStrictEqual(153)
Each seam comes with an enabling point: “a place where you can
make the decision to use one behavior or another” [WELC]. Passing the function as
parameter opens up an enabling point in the caller of
calculateShipping
.
This now makes testing a lot easier, we can put in different values of
shipping costs, and check that applyShippingDiscounts
responds
correctly. Although we had to change the original source code to introduce the
seam, any further changes to that function don't require us to alter that
code, the changes all occur in the enabling point, which lies in the test code.
Passing a function as a parameter isn't the only way we can introduce a
seam. After all, changing the signature of calculateShipping
may
be fraught, and we may not want to thread the shipping function parameter
through the legacy call stack in the production code. In this case a lookup
may be a better approach, such as using a service locator.
export async function calculatePrice(order:Order) {
const itemPrices = order.items.map(i => calculateItemPrice(i))
const basePrice = itemPrices.reduce((acc, i) => acc + i.price, 0)
const discount = calculateDiscount(order)
const shipping = await ShippingServices.calculateShipping(order)
const adjustedShipping = applyShippingDiscounts(order, shipping)
return basePrice + discount + adjustedShipping
}
class ShippingServices { static #soleInstance: ShippingServices static init(arg?:ShippingServices) { this.#soleInstance = arg || new ShippingServices() } static async calculateShipping(o:Order) {return this.#soleInstance.calculateShipping(o)} async calculateShipping(o:Order) {return legacy_calcuateShipping(o)} // ... more services
The locator allows us to override the behavior by defining a subclass.
class ShippingServicesStub extends ShippingServices { calculateShippingFn: typeof ShippingServices.calculateShipping = (o) => {throw new Error("no stub provided")} async calculateShipping(o:Order) {return this.calculateShippingFn(o)} // more services
We can then use an enabling point in our test
const stub = new ShippingServicesStub() stub.calculateShippingFn = async (o:Order) => 113 ShippingServices.init(stub) expect(await calculatePrice(sampleOrder)).toStrictEqual(153)
This kind of service locator is a classical object-oriented way to set up a seam via function lookup, which I'm showing here to indicate the kind of approach I might use in other languages, but I wouldn't use this approach in TypeScript or JavaScript. Instead I'd put something like this into a module.
export let calculateShipping = legacy_calculateShipping export function reset_calculateShipping(fn?: typeof legacy_calculateShipping) { calculateShipping = fn || legacy_calculateShipping }
We can then use the code in a test like this
const shippingFn = async (o:Order) => 113 reset_calculateShipping(shippingFn) expect(await calculatePrice(sampleOrder)).toStrictEqual(153)
As the final example suggests, the best mechanism to use for a seam depends very much on the language, available frameworks, and indeed the style of the legacy system. Getting a legacy system under control means learning how to introduce various seams into the code to provide the right kind of enabling points while minimizing the disturbance to the legacy software. While a function call is a simple example of introducing such seams, they can be much more intricate in practice. A team can spend several months figuring out how to introduce seams into a well-worn legacy system. The best mechanism for adding seams to a legacy system may be different to what we'd do for similar flexibility in a green field.
Feathers's book focuses primarily on getting a legacy system under test, as
that is often the key to being able to work with it in a sane way. But seams
have more uses than that. Once we have a seam, we are in the position to place
probes into the legacy system, allowing us to increase the observability of
the system. We might want to monitor calls to calculateShipping
,
figuring out how often we use it, and capturing its results for separate analysis.
But probably the most valuable use of seams is that they allow us to migrate behavior away from the legacy. A seam might redirect high-value customers to a different shipping calculator. Effective legacy displacement is founded on introducing seams into the legacy system, and using them to gradually move behavior into a more modern environment.
Seams are also something to think about as we write new software, after all every new system will become legacy sooner or later. Much of my design advice is about building software with appropriately placed seams, so we can easily test, observe, and enhance it. If we write our software with testing in mind, we tend to get a good set of seams, which is a reason why Test Driven Development is such a useful technique.
Software And Engineering
13 December 2023
Throughout my career, people have compared software development to “traditional” engineering, usually in a way to scold software developers for not doing a proper job. As someone who got his degree in Electronic Engineering, this resonated with me early in my career. But this way of thinking is flawed because most people have the wrong impression of how engineering works in practice.
Glenn Vanderburg has spent a lot of time digging into these misconceptions, and I strongly urge anyone who wants to compare software development to engineering to watch his talk Real Software Engineering. It's also well worth listening to his interview on the podcast Oddly Influenced. Sadly I've not been able to persuade him to write this material down - it would make a great article.
Another good thinker on this relationship is Hillel Wayne. He interviewed a bunch of “crossovers” - people who had worked both in traditional engineering and in software. He wrote up what he learned in a series of essays, starting with Are We Really Engineers?
Test Driven Development
11 December 2023
Test-Driven Development (TDD) is a technique for building software that guides software development by writing tests. It was developed by Kent Beck in the late 1990's as part of Extreme Programming. In essence we follow three simple steps repeatedly:
- Write a test for the next bit of functionality you want to add.
- Write the functional code until the test passes.
- Refactor both new and old code to make it well structured.
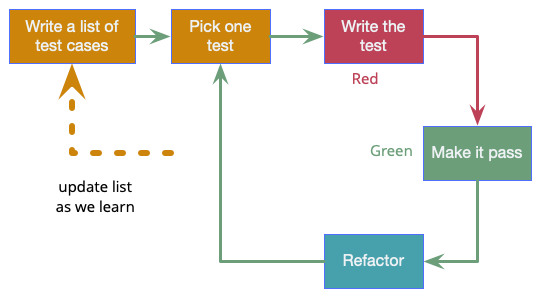
Although these three steps, often summarized as Red - Green - Refactor, are the heart of the process, there's also a vital initial step where we write out a list of test cases first. We then pick one of these tests, apply red-green-refactor to it, and once we're done pick the next. Sequencing the tests properly is a skill, we want to pick tests that drive us quickly to the salient points in the design. During the process we should add more tests to our lists as they occur to us.
Writing the test first, what XPE2 calls Test-First Programming, provides two main benefits. Most obviously it's a way to get SelfTestingCode, since we can only write some functional code in response to making a test pass. The second benefit is that thinking about the test first forces us to think about the interface to the code first. This focus on interface and how you use a class helps us separate interface from implementation, a key element of good design that many programmers struggle with.
The most common way that I hear to screw up TDD is neglecting the third step. Refactoring the code to keep it clean is a key part of the process, otherwise we just end up with a messy aggregation of code fragments. (At least these will have tests, so it's a less painful result than most failures of design.)
Further Reading
Kent's summary of the canonical way to do TDD is the key online summary.
For more depth, head to Kent Beck's book Test-Driven Development.
The relevant chapter of James Shore's The Art of Agile Development is another sound description that also connects it to the rest of effective agile development. James also wrote a series of screencasts called Let's Play TDD.
Revisions
My original post of this page was 2005-03-05. Inspired by Kent's canonical post, I updated it on 2023-12-11
Diff Debugging
4 December 2023
Regression bugs are newly appeared bugs in features of the software that have been around for a while. When hunting them, it usually valuable to figure out which change in the software caused them to appear. Looking at that change can give invaluable clues about where the bug is and how to squash it. There isn't a well-known term for this form of investigation, but I call it Diff Debugging.
Diff debugging only works if we have our code in version control, but fortunately these days that's the norm. But there are some more things that are needed to make it work effectively. We need Reproducible Builds, so that we can run old versions of the software easily. It helps greatly to have small commits, due to high-frequency integration. That way when we find the guilty commit, we can more easily narrow down what happened.
To find the commit that bred the bug, we begin by finding any past version without the bug. Mark this as a last-good version and the current version as the earliest-bad. Then find the commit half-way between the two and see if the bug is there. If so then this commit becomes the earliest-bad, otherwise it becomes the last-good. Repeat the process (which is a “half-interval” or “binary” search) until we've got the guilty commit.
If we use git, then the git bisect command will automate much of this for us. If we can write a test that will show the presence of the bug, then git bisect can use that too, automating the whole process of finding the guilty commit.
I often find diff debugging to be useful within a programming session. If I have slow tests that take a few minutes to run, I might program for half-an-hour running only a subset of the most relevant tests. As long as I commit after every green test run, I can use diff debugging should one of those slower tests fail. Such is the value of committing extremely frequently, even if they are so small that I feel its best to squash them for the long-term history. Some IDEs make this easier by keeping a local history automatically that is finer-grained than the commits to version control.
Revisions
I originally posted this page on 2004-06-01. In its original form it was more of a casual experience report. I rewrote it on 2023-12-04 to make it more like a definition of the term. Diff debugging isn't a term that's caught on much in the industry, but I haven't seen a another term generally used to describe it.