Periodic Face-to-Face
27 February 2024
Improvements in communications technology have led an increasing number of teams that work in a Remote-First style, a trend that was boosted by the forced isolation of Covid-19 pandemic. But a team that operates remotely still benefits from face-to-face gatherings, and should do them every few months.
Remote-first teams have everyone in a separate location, communicating entirely by email, chat, video and other communication tools. It has definite benefits: people can be recruited to the team from all over the world, and we can involve people with care-giving responsibilities. Wasteful hours of frustrating commutes can be turned into productive or recuperative time.
But however capable folks may be at remote working, and however nifty modern collaboration tools become, there is still nothing like being in the same place with the other members of a team. Human interactions are always richer when they are face-to-face. Video calls too easily become transactional, with little time for the chitchat that builds a proper human relationship. Without those deeper bonds, misunderstandings fester into serious relationship difficulties, and teams can get tangled in situations that would be effectively resolved if everyone were able to talk in person.
A regular pattern I see from those who are effective in remote-first work is that they ensure regular face-to-face meetings. During these they schedule those elements of work that are done better together. Remote work is more effective for tasks that require solo concentration, and modern tools can make remote pairing workable. But tasks that require lots of input from many people with rapid feedback are much easier to do when everyone is in the same room. No video-conference system can create the that depth of interaction, staring at a computer screen to see what other people are doing is draining, with no opportunity to pop out for a coffee together to break up the work. Debates about product strategy, explorations of systems architecture, explorations of new ground - these are common tasks for when the team is assembled.
For people to work effectively together they need to trust each other, aware of how much they can rely on each other. Trust is hard to develop online, where there isn't the social cues that can happen when we are in the same room. Thus the most valuable part of a face-to-face gathering isn't the scheduled work, it's chitchat while getting a coffee, and conviviality over lunch. Informal conversations, mostly not about work, forge the human contact that makes the work interactions be more effective.
Those guidelines suggest what the content for a face-to-face should be. Working together is both valuable in its own right, and an important part of team bonding. So we should set a full day of work, focusing on those tasks that benefit from the low-latency communication that comes from being together. We should then include what feels like too much time for breaks, informal chatter, and opportunities to step outside the office. I would avoid any artificial “team building” exercises, if only because of how much I hate them. Those who do gatherings like this stress the value from everyone energized afterwards, and thus able to be more effective in the following weeks.
Remote teams can be formed at large distances, and it's common to see members separated by hours of travel. For such teams, the rule of thumb I would use is to get together for a week every two or three months. After the team has become seasoned they may then decide to reduce the frequency, but I would worry if a team isn't having at least two face-to-face meetings a year. If a team is all in the same city, but using a remote-first style to reduce commuting, then they can organize shorter gatherings, and do them more frequently.
This kind of gathering may lead to rethinking of how to configure office space. Much has been made of how offices are far less used since the pandemic. Offices could well become less of a day-to-day workspace, and more a location for these kinds of irregular team gatherings. This leads to a need for flexible and comfortable team gathering spaces.
Some organizations may balk at the costs of travel and accommodation for a team assembly like this, but they should think of it as an investment in the team's effectiveness. Neglecting these face-to-faces leads to teams getting stuck, heading off in the wrong direction, plagued with conflict, and people losing motivation. Compared to this, saving on airplanes and hotels is a false economy.
Further Reading
Remote-first is one form of remote work, I explore the different styles of remote working and their trade-offs in Remote versus Co-located Work.
At Thoughtworks, we learned the importance of regular face-to-face gatherings for remote teams when we first started our offshore development centers nearly two decades ago. These generated the practices I describe in Using an Agile Software Process with Offshore Development.
Remote work, particularly when crossing time zones, puts a greater premium on asynchronous patterns of collaboration. My colleague Sumeet Moghe, a product manager, goes into depth on how to do this in his book The Async-First Playbook
Atlassian, a software product company, has recently entirely shifted to remote working, and published a report on its experiences. They have learned that it's wise for teams to have a face-to-face gathering roughly three times per year. Claire Lew surveyed remote-first teams in 2018, noting that a quarter of their respondents did retreats “several times a year”. 37Signals has operated as a remote-first company for nearly two decades and schedules meetups twice a year.
Acknowledgements
Alejandro Batanero, Andrew Thal, Chris Ford, Heiko Gerin, Kief Morris, Kuldeep Singh, Matt Newman, Michael Chaffee, Naval Prabhakar, Rafael Detoni, and Ramki Sitaraman discussed drafts of this post on our internal mailing list.
Legacy Seam
4 January 2024
When working with a legacy system it is valuable to identify and create seams: places where we can alter the behavior of the system without editing source code. Once we've found a seam, we can use it to break dependencies to simplify testing, insert probes to gain observability, and redirect program flow to new modules as part of legacy displacement.
Michael Feathers coined the term “seam” in the context of legacy systems in his book Working Effectively with Legacy Code. His definition: “a seam is a place where you can alter behavior in your program without editing in that place”.
Here's an example of where a seam would be handy. Imagine some code to calculate the price of an order.
// TypeScript
export async function calculatePrice(order:Order) {
const itemPrices = order.items.map(i => calculateItemPrice(i))
const basePrice = itemPrices.reduce((acc, i) => acc + i.price, 0)
const discount = calculateDiscount(order)
const shipping = await calculateShipping(order)
const adjustedShipping = applyShippingDiscounts(order, shipping)
return basePrice + discount + adjustedShipping
}
The function calculateShipping
hits an external service, which is slow (and
expensive), so we don't want to hit it when testing. Instead we want to
introduce a stub, so we can provide a canned and deterministic response for
each of the testing scenarios. Different tests may need different responses
from the function, but we can't edit the code of
calculatePrice
inside the test. Thus we need to introduce a seam around the call
to calculateShipping
, something that will allow our test to
redirect the call to the stub.
One way to do this is to pass the function for
calculateShipping
as a parameter
export async function calculatePrice(order:Order, shippingFn: (o:Order) => Promise<number>) { const itemPrices = order.items.map(i => calculateItemPrice(i)) const basePrice = itemPrices.reduce((acc, i) => acc + i.price, 0) const discount = calculateDiscount(order) const shipping = await shippingFn(order) const adjustedShipping = applyShippingDiscounts(order, shipping) return basePrice + discount + adjustedShipping }
A unit test for this function can then substitute a simple stub.
const shippingFn = async (o:Order) => 113 expect(await calculatePrice(sampleOrder, shippingFn)).toStrictEqual(153)
Each seam comes with an enabling point: “a place where you can
make the decision to use one behavior or another” [WELC]. Passing the function as
parameter opens up an enabling point in the caller of
calculateShipping
.
This now makes testing a lot easier, we can put in different values of
shipping costs, and check that applyShippingDiscounts
responds
correctly. Although we had to change the original source code to introduce the
seam, any further changes to that function don't require us to alter that
code, the changes all occur in the enabling point, which lies in the test code.
Passing a function as a parameter isn't the only way we can introduce a
seam. After all, changing the signature of calculateShipping
may
be fraught, and we may not want to thread the shipping function parameter
through the legacy call stack in the production code. In this case a lookup
may be a better approach, such as using a service locator.
export async function calculatePrice(order:Order) {
const itemPrices = order.items.map(i => calculateItemPrice(i))
const basePrice = itemPrices.reduce((acc, i) => acc + i.price, 0)
const discount = calculateDiscount(order)
const shipping = await ShippingServices.calculateShipping(order)
const adjustedShipping = applyShippingDiscounts(order, shipping)
return basePrice + discount + adjustedShipping
}
class ShippingServices { static #soleInstance: ShippingServices static init(arg?:ShippingServices) { this.#soleInstance = arg || new ShippingServices() } static async calculateShipping(o:Order) {return this.#soleInstance.calculateShipping(o)} async calculateShipping(o:Order) {return legacy_calcuateShipping(o)} // ... more services
The locator allows us to override the behavior by defining a subclass.
class ShippingServicesStub extends ShippingServices { calculateShippingFn: typeof ShippingServices.calculateShipping = (o) => {throw new Error("no stub provided")} async calculateShipping(o:Order) {return this.calculateShippingFn(o)} // more services
We can then use an enabling point in our test
const stub = new ShippingServicesStub() stub.calculateShippingFn = async (o:Order) => 113 ShippingServices.init(stub) expect(await calculatePrice(sampleOrder)).toStrictEqual(153)
This kind of service locator is a classical object-oriented way to set up a seam via function lookup, which I'm showing here to indicate the kind of approach I might use in other languages, but I wouldn't use this approach in TypeScript or JavaScript. Instead I'd put something like this into a module.
export let calculateShipping = legacy_calculateShipping export function reset_calculateShipping(fn?: typeof legacy_calculateShipping) { calculateShipping = fn || legacy_calculateShipping }
We can then use the code in a test like this
const shippingFn = async (o:Order) => 113 reset_calculateShipping(shippingFn) expect(await calculatePrice(sampleOrder)).toStrictEqual(153)
As the final example suggests, the best mechanism to use for a seam depends very much on the language, available frameworks, and indeed the style of the legacy system. Getting a legacy system under control means learning how to introduce various seams into the code to provide the right kind of enabling points while minimizing the disturbance to the legacy software. While a function call is a simple example of introducing such seams, they can be much more intricate in practice. A team can spend several months figuring out how to introduce seams into a well-worn legacy system. The best mechanism for adding seams to a legacy system may be different to what we'd do for similar flexibility in a green field.
Feathers's book focuses primarily on getting a legacy system under test, as
that is often the key to being able to work with it in a sane way. But seams
have more uses than that. Once we have a seam, we are in the position to place
probes into the legacy system, allowing us to increase the observability of
the system. We might want to monitor calls to calculateShipping
,
figuring out how often we use it, and capturing its results for separate analysis.
But probably the most valuable use of seams is that they allow us to migrate behavior away from the legacy. A seam might redirect high-value customers to a different shipping calculator. Effective legacy displacement is founded on introducing seams into the legacy system, and using them to gradually move behavior into a more modern environment.
Seams are also something to think about as we write new software, after all every new system will become legacy sooner or later. Much of my design advice is about building software with appropriately placed seams, so we can easily test, observe, and enhance it. If we write our software with testing in mind, we tend to get a good set of seams, which is a reason why Test Driven Development is such a useful technique.
Software And Engineering
13 December 2023
Throughout my career, people have compared software development to “traditional” engineering, usually in a way to scold software developers for not doing a proper job. As someone who got his degree in Electronic Engineering, this resonated with me early in my career. But this way of thinking is flawed because most people have the wrong impression of how engineering works in practice.
Glenn Vanderburg has spent a lot of time digging into these misconceptions, and I strongly urge anyone who wants to compare software development to engineering to watch his talk Real Software Engineering. It's also well worth listening to his interview on the podcast Oddly Influenced. Sadly I've not been able to persuade him to write this material down - it would make a great article.
Another good thinker on this relationship is Hillel Wayne. He interviewed a bunch of “crossovers” - people who had worked both in traditional engineering and in software. He wrote up what he learned in a series of essays, starting with Are We Really Engineers?
Test Driven Development
11 December 2023
Test-Driven Development (TDD) is a technique for building software that guides software development by writing tests. It was developed by Kent Beck in the late 1990's as part of Extreme Programming. In essence we follow three simple steps repeatedly:
- Write a test for the next bit of functionality you want to add.
- Write the functional code until the test passes.
- Refactor both new and old code to make it well structured.
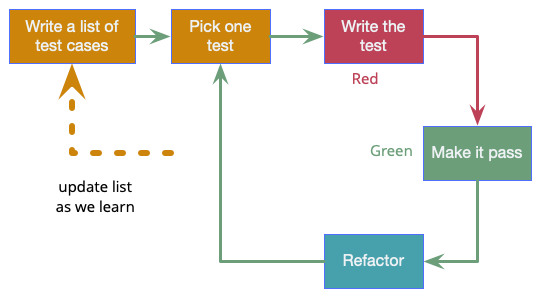
Although these three steps, often summarized as Red - Green - Refactor, are the heart of the process, there's also a vital initial step where we write out a list of test cases first. We then pick one of these tests, apply red-green-refactor to it, and once we're done pick the next. Sequencing the tests properly is a skill, we want to pick tests that drive us quickly to the salient points in the design. During the process we should add more tests to our lists as they occur to us.
Writing the test first, what XPE2 calls Test-First Programming, provides two main benefits. Most obviously it's a way to get SelfTestingCode, since we can only write some functional code in response to making a test pass. The second benefit is that thinking about the test first forces us to think about the interface to the code first. This focus on interface and how you use a class helps us separate interface from implementation, a key element of good design that many programmers struggle with.
The most common way that I hear to screw up TDD is neglecting the third step. Refactoring the code to keep it clean is a key part of the process, otherwise we just end up with a messy aggregation of code fragments. (At least these will have tests, so it's a less painful result than most failures of design.)
Further Reading
Kent's summary of the canonical way to do TDD is the key online summary.
For more depth, head to Kent Beck's book Test-Driven Development.
The relevant chapter of James Shore's The Art of Agile Development is another sound description that also connects it to the rest of effective agile development. James also wrote a series of screencasts called Let's Play TDD.
Revisions
My original post of this page was 2005-03-05. Inspired by Kent's canonical post, I updated it on 2023-12-11
Diff Debugging
4 December 2023
Regression bugs are newly appeared bugs in features of the software that have been around for a while. When hunting them, it usually valuable to figure out which change in the software caused them to appear. Looking at that change can give invaluable clues about where the bug is and how to squash it. There isn't a well-known term for this form of investigation, but I call it Diff Debugging.
Diff debugging only works if we have our code in version control, but fortunately these days that's the norm. But there are some more things that are needed to make it work effectively. We need Reproducible Builds, so that we can run old versions of the software easily. It helps greatly to have small commits, due to high-frequency integration. That way when we find the guilty commit, we can more easily narrow down what happened.
To find the commit that bred the bug, we begin by finding any past version without the bug. Mark this as a last-good version and the current version as the earliest-bad. Then find the commit half-way between the two and see if the bug is there. If so then this commit becomes the earliest-bad, otherwise it becomes the last-good. Repeat the process (which is a “half-interval” or “binary” search) until we've got the guilty commit.
If we use git, then the git bisect command will automate much of this for us. If we can write a test that will show the presence of the bug, then git bisect can use that too, automating the whole process of finding the guilty commit.
I often find diff debugging to be useful within a programming session. If I have slow tests that take a few minutes to run, I might program for half-an-hour running only a subset of the most relevant tests. As long as I commit after every green test run, I can use diff debugging should one of those slower tests fail. Such is the value of committing extremely frequently, even if they are so small that I feel its best to squash them for the long-term history. Some IDEs make this easier by keeping a local history automatically that is finer-grained than the commits to version control.
Revisions
I originally posted this page on 2004-06-01. In its original form it was more of a casual experience report. I rewrote it on 2023-12-04 to make it more like a definition of the term. Diff debugging isn't a term that's caught on much in the industry, but I haven't seen a another term generally used to describe it.
Team Topologies
25 July 2023
Any large software effort, such as the software estate for a large company, requires a lot of people - and whenever you have a lot of people you have to figure out how to divide them into effective teams. Forming Business Capability Centric teams helps software efforts to be responsive to customers’ needs, but the range of skills required often overwhelms such teams. Team Topologies is a model for describing the organization of software development teams, developed by Matthew Skelton and Manuel Pais. It defines four forms of teams and three modes of team interactions. The model encourages healthy interactions that allow business-capability centric teams to flourish in their task of providing a steady flow of valuable software.
The primary kind of team in this framework is the stream-aligned team, a Business Capability Centric team that is responsible for software for a single business capability. These are long-running teams, thinking of their efforts as providing a software product to enhance the business capability.
Each stream-aligned team is full-stack and full-lifecycle: responsible for front-end, back-end, database, business analysis, feature prioritization, UX, testing, deployment, monitoring - the whole enchilada of software development. They are Outcome Oriented, focused on business outcomes rather than Activity Oriented teams focused on a function such as business analysis, testing, or databases. But they also shouldn't be too large, ideally each one is a Two Pizza Team. A large organization will have many such teams, and while they have different business capabilities to support, they have common needs such as data storage, network communications, and observability.
A small team like this calls for ways to reduce their cognitive load, so they can concentrate on supporting the business needs, not on (for example) data storage issues. An important part of doing this is to build on a platform that takes care of these non-focal concerns. For many teams a platform can be a widely available third party platform, such as Ruby on Rails for a database-backed web application. But for many products there is no single off-the-shelf platform to use, a team is going to have to find and integrate several platforms. In a larger organization they will have to access a range of internal services and follow corporate standards.
This problem can be addressed by building an internal platform for the organization. Such a platform can do that integration of third-party services, near-complete platforms, and internal services. Team Topologies classifies the team that builds this (unimaginatively-but-wisely) as a platform team.
Smaller organizations can work with a single platform team, which produces a thin layer over an externally provided set of products. Larger platforms, however, require more people than can be fed with two-pizzas. The authors are thus moving to describe a platform grouping of many platform teams.
An important characteristic of a platform is that it's designed to be used in a mostly self-service fashion. The stream-aligned teams are still responsible for the operation of their product, and direct their use of the platform without expecting an elaborate collaboration with the platform team. In the Team Topologies framework, this interaction mode is referred to as X-as-a-Service mode, with the platform acting as a service to the stream-aligned teams.
Platform teams, however, need to build their services as products themselves, with a deep understanding of their customer's needs. This often requires that they use a different interaction mode, one of collaboration mode, while they build that service. Collaboration mode is a more intensive partnership form of interaction, and should be seen as a temporary approach until the platform is mature enough to move to x-as-a service mode.
So far, the model doesn't represent anything particularly inventive. Breaking organizations down between business-aligned and technology support teams is an approach as old as enterprise software. In recent years, plenty of writers have expressed the importance of making these business capability teams be responsible for the full-stack and the full-lifecycle. For me, the bright insight of Team Topologies is focusing on the problem that having business-aligned teams that are full-stack and full-lifecycle means that they are often faced with an excessive cognitive load, which works against the desire for small, responsive teams. The key benefit of a platform is that it reduces this cognitive load.
A crucial insight of Team Topologies is that the primary benefit of a platform is to reduce the cognitive load on stream-aligned teams
This insight has profound implications. For a start it alters how platform teams should think about the platform. Reducing client teams' cognitive load leads to different design decisions and product roadmap to platforms intended primarily for standardization or cost-reduction. Beyond the platform this insight leads Team Topologies to develop their model further by identifying two more kinds of team.
Some capabilities require specialists who can put considerable time and energy into mastering a topic important to many stream-aligned teams. A security specialist may spend more time studying security issues and interacting with the broader security community than would be possible as a member of a stream-aligned team. Such people congregate in enabling teams, whose role is to grow relevant skills inside other teams so that those teams can remain independent and better own and evolve their services. To achieve this enabling teams primarily use the third and final interaction mode in Team Topologies. Facilitating mode involves a coaching role, where the enabling team isn't there to write and ensure conformance to standards, but instead to educate and coach their colleagues so that the stream-aligned teams become more autonomous.
Stream-aligned teams are responsible for the whole stream of value for their customers, but occasionally we find aspects of a stream-aligned team's work that is sufficiently demanding that it needs a dedicated group to focus on it, leading to the fourth and final type of team: complicated-subsystem team. The goal of a complicated-subsystem team is to reduce the cognitive load of the stream-aligned teams that use that complicated subsystem. That's a worthwhile division even if there's only one client team for that subsystem. Mostly complicated-subsystem teams strive to interact with their clients using x-as-a service mode, but will need to use collaboration mode for short periods.
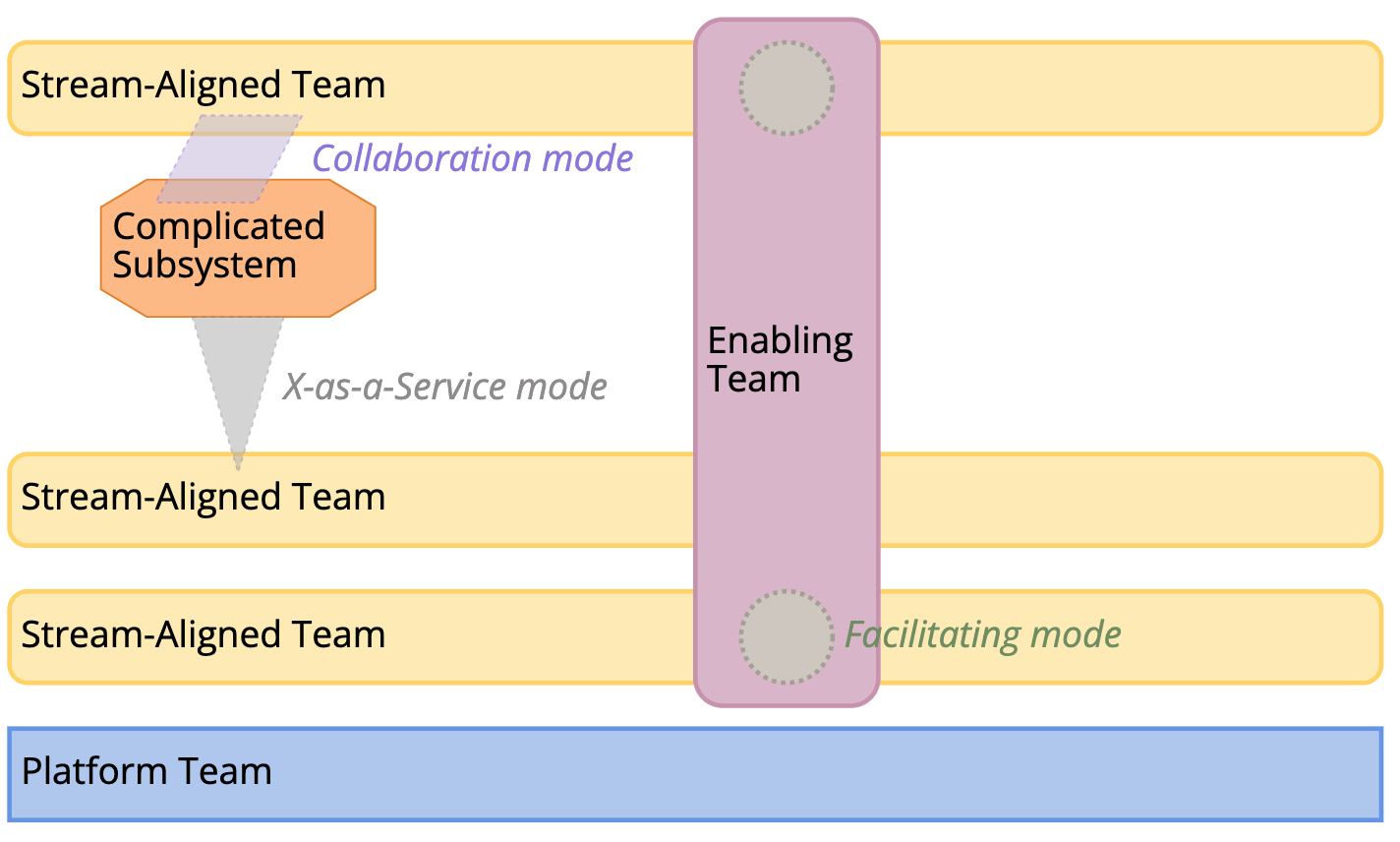
Team Topologies includes a set of graphical symbols to illustrate teams and their relationships. These shown here are from the current standards, which differ from those used in the book. A recent article elaborates on how to use these diagrams.
Team Topologies is designed explicitly recognizing the influence of Conways Law. The team organization that it encourages takes into account the interplay between human and software organization. Advocates of Team Topologies intend its team structure to shape the future development of the software architecture into responsive and decoupled components aligned to business needs.
George Box neatly quipped: "all models are wrong, some are useful". Thus Team Topologies is wrong: complex organizations cannot be simply broken down into just four kinds of teams and three kinds of interactions. But constraints like this are what makes a model useful. Team Topologies is a tool that impels people to evolve their organization into a more effective way of operating, one that allows stream-aligned teams to maximize their flow by lightening their cognitive load.
Acknowledgements
Andrew Thal, Andy Birds, Chris Ford, Deepak Paramasivam, Heiko Gerin, Kief Morris, Matteo Vaccari, Matthew Foster, Pavlo Kerestey, Peter Gillard-Moss, Prashanth Ramakrishnan, and Sandeep Jagtap discussed drafts of this post on our internal mailing list, providing valuable feedback.
Matthew Skelton and Manuel Pais kindly provided detailed comments on this post, including sharing some of their recent thinking since the book.
Further Reading
The best treatment of the Team Topologies framework is the book of the same name, published in 2019. The authors also maintain the Team Topologies website and provide education and training services. Their recent article on team interaction modeling is a good intro to how the Team Topologies (meta-)model can be used to build and evolve a model of an organization. [1]
Much of Team Topologies is based on the notion of Cognitive Load. The authors explored cognitive load in Tech Beacon. Jo Pearce expanded on how cognitive load may apply to software development.
The model in Team Topologies resonates well with much of the thinking on software team organization that I've published on this site. You can find this collected together at the team organization tag.
Notes
1: To be more strict in my modeling lingo, I would say that Team Topologies usually acts as a meta-model. If I use Team Topologies to build a model of an airline's software development organization, then that model shows the teams in the airline classified according to Team Topologies's terminology. I would then say that that the Team Topologies model is a meta-model to my airline model.
Two Pizza Team
25 July 2023
A two-pizza team is a small team that fully supports software for a particular business capability. The term became popular as it used to describe how Amazon organized their software staff.
The name suggests the most obvious aspect of such teams, their size. The name comes from the principle that the team should no larger than can be fed with two pizzas. (Although we are talking about American Pizzas here, which seemed alarmingly huge when I first encountered them over here.) Keeping a team small keeps it cohesive, forming tight working relationships. Typically I hear this means such teams are about 5-8 people, although my experience suggests that the upper limit is somewhere about 15.
Although the name focuses solely on the size, just as important is the team's focus. A two-pizza team should have all the capabilities it needs to delivery valuable software to its users, with minimal hand-offs and dependencies on other teams. They can figure out what their customer needs, and quickly translate that into working software, able to experiment and evolve that software as their customer's needs change.
Two-pizza teams are Outcome Oriented rather than Activity Oriented. They don't organize along lines of skills (databases, testing, operations), instead they take on all the responsibilities required to support their customers. This minimizes inter-team hand-offs in the flow of features to their customers, allowing them to reduce the cycle-time (the time required to turn an idea for a feature into code running in production). This outcome-orientation also means they deploy code into production and monitor its use there, famously responsible for any production outages (often meaning they on the hook for off-hours support) - a principle known as "you build it, you run it".
Focusing on a customer need like this means teams are long-lived, Business Capability Centric teams that support their business capability as long as that capability is active. Unlike project-oriented teams - that disband when the software is "done" - they think of themselves as enabling and enhancing a long-lived product. This aspect often leads to them being referred to as product teams.
The wide scope of skills and responsibilities that a two-pizza team needs to support its product means that although such teams can be the primary approach to team organization, they need support from a well-constructed software platform. For small organizations, this can be a commercial platform, such as a modern cloud offering. Larger organizations will create their own internal platforms to make it easier for their two-pizza teams to collaborate without creating difficult hand-offs. Team Topologies provides a good way to think about the different kinds of teams and interactions required to support two-pizza teams (Team Topologies calls them stream-aligned teams).
For business-capability centric teams to be effective, they will need to make use of each others' capabilities. Teams will thus need to provide their capabilities to their peers, often though thoughtfully designed APIs. This responsibility for such teams to provide services to their peers is often overlooked, if it doesn't happen it will lead to sclerotic information silos.
Organizing people around business capabilities like this has a profound interaction with the way the software for an organization is structured - due to the effect of Conways Law. Software components built by two-pizza teams need well-controlled interactions with their peers, with clear APIs between them. This thinking led to the development of microservices, but that's not the only approach - well-structured components within a monolithic run-time is often a better path.